Data-driven Banking in Practice
More insights through analytics and data enrichment
More insights through analytics and data enrichment
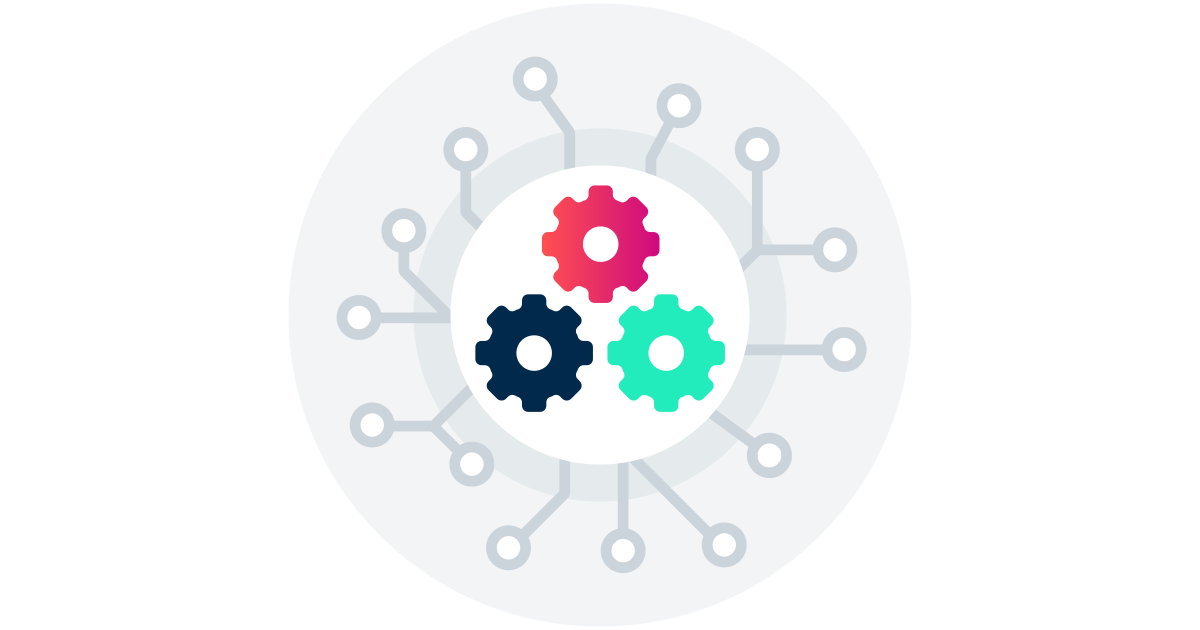
Data-driven Banking in Practice
Digitalisation is revolutionising one industry after another. It is now also opening up new opportunities for the Swiss financial sector. Under the motto of data-driven banking, banks are increasingly leveraging data to improve their processes, boost efficiency, and offer forward-looking, customer-centric digital service innovations.
The first requirement, however, is for the available data to be effectively and reliably recorded, analysed, and then enriched with additional relevant information. This challenging process is made possible with modern analytics solutions, which are also offered by specialised third-party providers such as Contovista (Contovista Enrichment Engine).
Such technologies, for example those based on artificial intelligence (AI), lay the foundations for the digital revolution in banking. Below you can read an overview of the most important aspects of data-driven banking: what it brings, how it works, what hurdles need to be overcome, and how it is implemented in practice.
- Data, Data, Data: Why Data-Driven Banking Is the Future
- From Customer Management to Compliance: The Potential of Data-Driven Banking
- From Silo Thinking to Data Protection: Challenges and Hurdles for Swiss Banks
- Using Transaction Data – with the Contovista Enrichment Engine
- Conclusion and Outlook: Data is the Future of Banking
1. Data, Data, Data: Why Data-Driven Banking Is the Future
Data-driven banking, which is based on the enrichment and analysis of transaction data, is becoming increasingly significant. Neobanks are leading the way here, but traditional banks can catch up by using anonymised data and their positioning to remain competitive and increase their profits.
The rise of online shopping, smartphone apps, and connected devices means that consumers today are generating more data than ever before, with this amount continuing to grow. In virtually all industries, this information is being used by companies to learn more about their customers and their needs – and then to serve them with innovative, personalised offerings.
This digital revolution is now also arriving in the banking sector, where customers have increasingly high digital expectations of banking services. New competitors such as FinTechs and neo-banks are already rolling out corresponding services. There are several reasons why they have a clear advantage over established banks:
- Digital infrastructure: Neobanks are designed as digital platforms from the outset, enabling data to be recorded, stored, and processed more efficiently.
- Agility and innovation: New providers are often more open to innovation and more agile in adapting to new tools and systems, such as data analytics and machine learning. Business models and structures are often more flexible.
- Customer centricity: Neobanks are strongly focused on the customer experience from the very outset and offer data-driven, personalised services.Fewer legacy problems: Unlike traditional banks, neobanks usually start from scratch and so do not have legacy systems and structures that could hinder fast and efficient data usage.
- Data protection and security: Neobanks often place great emphasis on data protection and security.
Established institutions are therefore under strong pressure to create digital offerings as well. However, their starting conditions are excellent, as these banks have an extraordinarily comprehensive and meaningful database at their disposal: the verified account and transaction information of bank customers.
In addition, customers place a high degree of trust in established banks, which is an essential prerequisite for their consent to use their data. There are typically long-standing customer relationships with personal contact via the branch network. Established banks have been able to distinguish themselves as an experienced, competent, and stable partner for their customers by providing personalised advice, even on complex issues. At the same time, they have greater financial resources and therefore more investment opportunities than neobanks. In addition, they offer a broader range of products and services that they can market using data-driven approaches.
Data-driven approaches are ushering in a new era in the financial sector. Established banks should now urgently position themselves for this and capitalise on their own strengths. As a recent study by the University of Lucerne (German) showed, they can benefit from a vast amount of untapped potential through data-driven banking, from reducing costs and minimising risk to increasing sales. Using data-driven, customer-centric offerings, the approach creates new areas of revenue, improves customer loyalty, and strengthens established banks in competition with neo-banks & co.
The data revolution is paying dividends! According to Accenture, digitally mature institutions achieve an average return on investment of 61 percent. Furthermore, the move towards data-driven banking is helping to drive forward digital transformation and the necessary cultural change within an organisation.
2. From Customer Management to Compliance: The Potential of Data-Driven Banking
Data-driven banking offers banks a huge amount of potential: they can improve customer relationships, develop innovative products, and tap into new areas of revenue. The approach also boasts strong compliance and optimises process efficiency, e.g. through automation.
With their customers’ account information, banks are sitting on a real treasure trove of data. There is a huge range of possible use cases for this, provided bank customers consent to their data being used. However, this shouldn’t pose a problem as long as providers can make customers beneficial and attractive data-driven offers that are relevant to their everyday lives. As a result, banks will reap the benefits of increased efficiency, lower costs, better risk management, and new revenue opportunities.
Here are some of the benefits of data-driven banking:
- Optimise the customer relationship
Data-driven target group analysis enables personalised offers both online and offline (e.g. by advisors in the branch). Information on purchasing behaviour, card usage, salary, geo-data, and life events such as house building or retirement are taken into account. This allows the bank to provide optimised offers at the right time and leverage cross-selling opportunities. Customer loyalty is increased and its role as the main bank is strengthened. - Develop new products and services
Data-driven banking lays the foundation for product innovations such as a digital finance manager for personalised everyday banking or a business customer financial cockpit with value-added features. New data-driven services in the area of sustainability are of particular interest in light of climate change and the rising demand for such services. - Increase efficiency, drive growth
Data-driven banking increases the level of automation and scalability, contributing to both cost reductions and revenue growth. Flexibility and efficiency are enhanced, especially in areas like sales or creditworthiness assessment processes. Approval processes for credit card limits or small loans can also be automated. - Improve risk management
Data insights in credit risk assessment help with risk management, e.g. through data on payment transactions to and from third-party banks or on purchasing behaviour and recurring payments. - Strengthen compliance
Data-driven banking enables more efficient KYC (Know Your Customer) thanks to data enrichment on topics such as third party providers, debt collection, and gambling. Anti-money laundering (AML) monitoring is also improved.
3. From Silo Thinking to Data Protection: Challenges and Hurdles for Swiss Banks
Banks have to overcome a number of challenges when implementing data-driven banking. For example, silos within the organisation often hinder innovation and there is typically a lack of digital expertise. In addition, ensuring data quality is very demanding, i.e. achieving a high hit rate when analysing transaction data. New regulatory provisions on data protection in Switzerland must also be observed.
The benefits of data-driven banking are obvious. However, many institutions are only just beginning to implement it. According to Accenture, 80 to 85 percent of companies in the financial sector remain stuck at the proof of concept stage on their journey towards data maturity. Many banks still have a number of challenges to overcome on the way to achieving seamless digital processes. Both organisational and technological aspects need to be considered.
There is often a lack of sufficient digital expertise and corresponding resources in companies. The current situation on the labour market makes it difficult to bridge skills gaps. On top of this, there are the challenges posed by widespread silo thinking and behaviour in organisations, as expert Ilario Giordanelli (Contovista Product Manager and Data Scientist) explains in this article. Over time, specialised departments have emerged in banks, making the overarching, cross-functional approach required for data-driven banking more difficult. Topics and initiatives are viewed in isolation, there is a lack of structures and standards for centralised data utilisation, or existing data governance hinders this process.
The challenge of data quality: The long-tail effect in transaction data analytics
However, there are also challenging hurdles to overcome on a technological level. For instance, insufficient preparation and enrichment of data often hinder digital innovation. The existing transaction data frequently consists of inconsistent text components, while payment terminals lack systematic labelling. As a result, payment information is often incomplete or incorrect. This makes it difficult to categorise merchant names and merchant categories. There are various reasons for this:
- Different sources and standards: Card transaction data often comes from different sources such as banks, payment systems, and merchants, which may use different standards and formats. This leads to inconsistencies and irregularities in the data.
- Limited control over data entry: Merchants and customers have limited control over the way transaction data is captured. For example, automated systems such as payment terminals can capture data in a way that is not always ideal for subsequent data analytics.
- Historical and technical limitations: Older payment systems often had limitations in terms of data formatting and storage. Sometimes these systems are still in use, or their data formats are retained for compatibility reasons.
When ensuring an appropriate quality of data analysis, these factors lead to a “long-tail effect”. This refers to the specific statistical distribution when categorising transaction data. The long-tail effect is of central importance for the hit rate. While the leading merchants account for the majority of market transactions and are therefore relatively easy to categorise using standard methods, categorising the numerous smaller market participants that form the long tail of the market poses a far greater challenge.
This is due to irregularities or peculiarities of the data sets (rare or unique transaction types, rarely used formats or encodings, etc.). These smaller players contribute significantly to market volume as a whole, although the complexity of categorising them increases exponentially as they represent a more diverse and specific range of transactions. While 75 percent coverage is still relatively easy to achieve, the effort required for the remaining 25 percent rises exponentially (see figure). However, these 25 percent are crucial for gaining comprehensive, reliable knowledge.
The quality and accuracy of categorisation is often critical as it can potentially provide vital insights. Errors in the categorisation of this data can lead to misleading analyses or decisions. However, processing this data is particularly resource-intensive, both in terms of human labour and technological resources. This is because this analysis often requires specialised algorithms and manual verification.
Figure: The long-tail effect in transaction data analysis.
The challenge of data protection: Current innovations in Swiss regulation
Another important challenge on the journey towards data-driven banking is data protection – a matter of fundamental importance for the success of the approach. When it comes to the personal data of clients (client identifying data, CID), the highest standards are of course necessary. This is the only way for users to place the necessary trust in innovative services. Moreover, this is the only way that banks also comply with extensive regulatory provisions regarding data protection.
A lot has happened recently in Switzerland. The new Data Protection Act (revDSG) has been in force since September 2023. It is somewhat similar to the EU’s General Data Protection Regulation (GDPR), although it does introduce a number of new requirements. Protection now only applies to natural persons, rather than legal entities. Certain obligations may arise for companies, such as order data processing contracts (ADV) or data protection impact assessments (DPIA).
Since data-driven banking is often implemented in the form of cloud-based Software-as-a-Service (SaaS) solutions, the new provisions on the cloud are of particular relevance, especially when it comes to the physical outsourcing of services. Data processing is only permitted in countries with an appropriate level of protection, which does not include the USA, for example. When selecting a technology provider, this aspect must be carefully considered; local solutions have a benefit here. With Contovista’s data-driven banking offering, the Enrichment Engine, all data remains in Switzerland (as SaaS data on Swiss servers or even on premise on the bank’s own servers).
4. Using Transaction Data – with the Contovista Enrichment Engine
External tools such as the Contovista Enrichment Engine provide an efficient solution to the challenges of data-driven banking. They are quick and easy to implement, provide high hit rates, strong data refinement, and allow personalised offers to be rolled out based on high-quality data. They already have valuable analytics tools on board.
Great opportunities, diverse challenges: data-driven banking is currently at the top of the agenda for Swiss banks. The task now is to break down existing silos and raise the standard for dealing with customers to a new level – from “Know Your Customer” (KYC) to “Understand Your Customer” (UYC).
The development of relevant skills and in-house resources such as data scientists and analytics is often challenging or too resource-intensive for many banks. This is particularly true if the aim is to ensure high quality, as the above example of the long-tail effect in categorisation illustrates. Against this backdrop, using a solution for transaction data enrichment from third-party providers, such as Contovista, is a viable option. Using the Contovista Enrichment Engine (EE) tool, we will now illustrate how the actual implementation works.
Interview with Eliane Albisser (Head of Product at Contovista)
Transaction data analytics with the Contovista Enrichment Engine
- Data refinement through AI analysis: In order to use transaction data in a meaningful way, it is first categorised by the Enrichment Engine. The merchant is identified using the unstructured raw data. AI algorithms can be used to derive the type of purchase or payment involved. The Enrichment Engine has an accuracy rate of over 98 percent when it comes to categorisation. Over 240 transaction categories such as “petrol station” or “supermarket” and over 13,500 merchant identities (with real names/“pretty names” and over 8,000 logos) are currently available. All common payment methods are recognised. The geopositioning of physical transactions is also carried out using open source data, for which Google services or similar are not required. The counterparty category is also recorded (counterparty category, over 1,000 categories that make it possible, for example, to differentiate between a pharmacy and a chemist or to identify payment transactions to people in the same household). The data set is then enriched by the Enrichment Engine.
- Data analysis with client analytics: In the next step, valuable insights can be derived from the enriched data. The Enrichment Engine provides users with fifteen pre-configured client analytics features out of the box – a unique offering on the Swiss market to date. These features assist in understanding the individual financial situation of clients, identifying important trends, and assessing financial health. Regular incoming and outgoing payments are recognised while expected future transactions are predicted. Information and analysis on third-party assets, annual salary, and bonuses are also recognised, along with major life events (change of employer, inheritance, retirement, etc.). An identity assessment sheds light on individual habits and preferences (e.g. travel behaviour, price sensitivity, sustainability).
- Leverage data through personalised offers: The results of the analysis can be fed into customer advisor systems, allowing banks to organise sales more efficiently through automated segmentation or automate credit approval processes. An easy-to-use REST API is available for integration into various existing solutions independent of the core banking system. The data can also be used for your own purposes, e.g. for training models or further use in digital channels. To make it even easier to leverage the underlying data for personalised products and services, Contovista offers a personal finance cockpit (Personal Finance Manager, PFM) that supports customers with their financial planning. Another option for private customers is the carbon footprint calculation, which draws on climate data from cooperation partner Deedster. For business customers, the Business Finance Manager (BFM) is available with options for liquidity planning, among other things.
- Continuous improvement: In order to capture changes in the retailer landscape and ensure the quality level of data enrichment, data and models must be constantly updated and maintained. To this end, open source data sources are used in our data centre together with state-of-the-art technology such as generative AI. The model improvement is carried out through the evaluation of non-customer identifying data (CID) by an AI-based auto-calibrator in the Contovista data centre. The process also involves quality assurance by domain experts, where validated updates are subsequently integrated back into the EE model (human-in-the-loop).
Figure: Transaction data analysis using the Contovista Enrichment Engine.
5. Platform effect through shared learning: The ongoing optimisation of the accuracy of transaction data refinement is driven even further by the inclusion of all partner banks (+25 banks so far). The non-CID data forwarded by them to the data centre is mutually complementary. For example, a bank in region A benefits from information on a merchant in region B, even if it was recorded there by another bank. As a result, the coverage and thus the hit rate increases for all parties involved. Since the data does not contain any customer-identifying information, data protection is guaranteed at all times.
Schematic illustration of the shared learning effect.
6. Security, data protection, and compliance at the highest level: The solution meets all regulatory requirements, such as the new Data Protection Act. The transaction enrichment service also fulfils Mastercard’s requirements to provide additional merchant information. Banks can choose between two implementation models: secure SaaS implementation via an API or an on-premise solution where no CID data leaves the data centre.
5. Conclusion and Outlook: Data Is the Future of Banking
Data-driven banking based on transaction data analysis enables banks to enter the world of personalised, customer-centric banking. By adopting this approach, banks are accelerating their digital transformation and continuously benefiting from the new capabilities that will be released in this dynamic field of technology in the coming years.
By analysing and refining transaction data, banks are taking a major step into the digital future. In all likelihood, however, this is just the beginning of the success story of data-driven banking. There are many other exciting future trends already on the horizon:
- New AI applications: An increasing number of innovative use cases are emerging in relation to AI, with generative AI opening up completely new dimensions. For example, high-quality personalised financial advice is made possible by bots or predictive analytics to enable more precise sales, which is also increasingly based on real-time data.
- The world of Open Banking: Multibanking enables the integration of various accounts and, consequently, the associated data. Insights into all customer accounts further increase the benefits of transaction data enrichment and analysis. Bank customers can now also increasingly leverage offerings from third-party providers. In Switzerland, there is currently noticeable movement in the field of multibanking, driven by initiatives from both the government and financial service providers, such as the bLink platform.
- The digital ecosystem of tomorrow: Data-driven banking, multibanking, and Open Banking are gradually creating a dynamic financial ecosystem. Alongside banks, other companies from sectors such as insurance and pensions will also participate, creating additional cross-selling opportunities, not to mention start-ups and financial service providers of all kinds. The result? More data and insights, new services and products, more customer-centric offerings, and a better user experience.
As you can see, the prospects for data-driven banking are bright. With Contovista’s Enrichment Engine, a powerful offering is available that banks can utilise to take the first step towards this future – simple, efficient, and fast. With Contovista, financial institutions are opting for a proven provider that understands the Swiss market inside out. Our Enrichment Engine offers leading technologies based on state-of-the-art AI and unique features, for example in the area of client analytics, so that banks can provide their customers with customised offers with lasting relevance – true to Contovista’s motto: Understand. Coach. Enable.